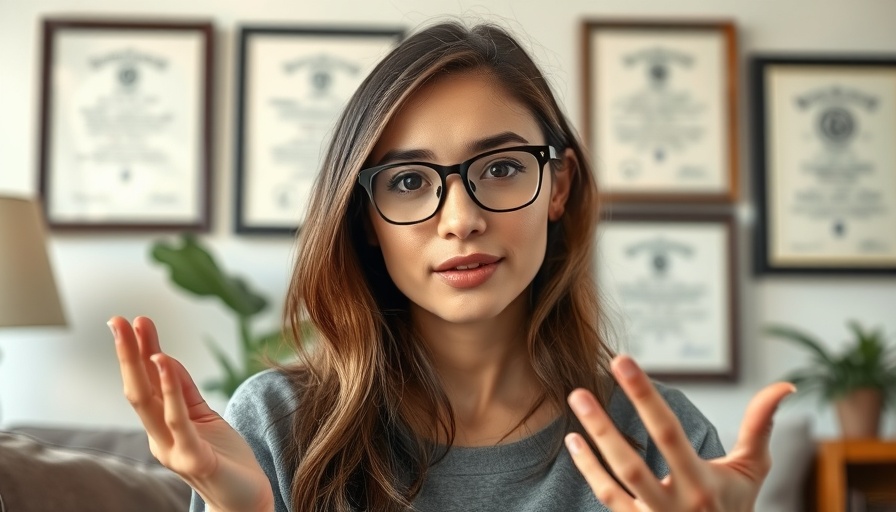
Understanding the Value of Synthetic Data
Synthetic data, a term gaining traction in the fields of technology and data science, serves as a powerful tool that can mimic real-world data without compromising privacy. This type of data can be generated through various methods that aim to either obscure sensitive information or create entirely new datasets based on existing patterns. While synthetic data has found its applications across industries, it is essential to recognize its limitations and the contexts in which it should be utilized.
In 'Understanding Synthetic Data When and Why It Matters,' the discussion dives into this complex but pivotal topic, exploring key insights that sparked deeper analysis on our end.
The Importance of Context in Data Usage
When employing synthetic data, one critical point of caution is its preliminary role in training machine learning models. While it can provide a significant advantage by offering a depth of data without personal identifiable information (PII), it is crucial to validate results against real-world datasets. Relying solely on synthetic data can lead to misguided assumptions that may propagate errors in analysis and decision-making.
Future Trends in Data Generation
As we anticipate the future of data generation, advancements in machine learning techniques promise to enhance the accuracy and utility of synthetic datasets. These improvements could lead to broader acceptance of synthetic data in applications ranging from autonomous vehicles to healthcare simulations—however, transparency in its origin and validation remains paramount. It is through these methods that synthetic data can step out of the realm of theoretical applications and evolve into a staple in modern data practices.
Write A Comment