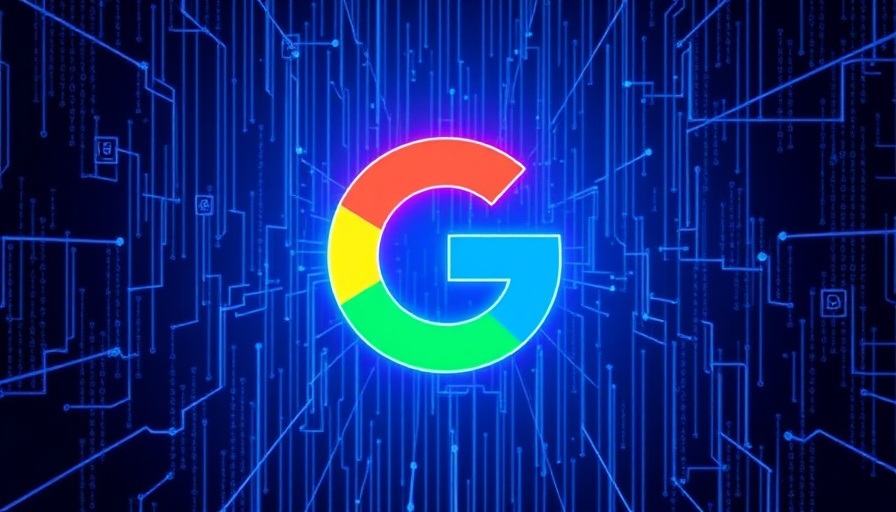
Introduction: Elevating AI Responses with Sufficient Context
Recent advancements in Artificial Intelligence (AI) have shifted toward enhancing the accuracy and reliability of responses generated by models such as Google's Gemini and OpenAI’s GPT. Google researchers have taken a significant step forward by refining Retrieval-Augmented Generation (RAG) models. This innovation centers around the introduction of a concept known as sufficient context, a principle designed to curb instances of hallucinations—where the AI generates potentially misleading or incorrect information.
What is Sufficient Context?
Sufficient context is defined as the presence of adequate details within the retrieved information that allows a model to derive a correct answer. However, it does not entail verifying the correctness of the answer itself but merely assessing whether a plausible response can be formulated from the given information. The lack of sufficient context typically indicates that the information is incomplete or missing critical details essential for providing an accurate answer.
Understanding the Hallucination Problem
AI models like Gemini and GPT often attempt to address queries without adequate context, leading to hallucinations instead of avoiding incorrect outputs altogether. Google's latest research reveals that proprietary models excel at delivering accurate answers when sufficient context is provided. Surprisingly, these models may still respond incorrectly 35-65% of the time when context falls short. This presents a dual challenge: discerning when to enable the model to answer and when to intervene to prevent inaccuracies.
Introducing the Sufficient Context Autorater
A breakthrough component of Google's findings is the Sufficient Context Autorater—an advanced system that classifies query-context pairs based on their contextual sufficiency. The most effective model, Gemini 1.5 Pro, achieved an impressive 93% accuracy, outperforming its peer models. This autorater significantly enhances the capability of RAG models by providing clarity on when contextual information is adequate.
Selective Generation: A Practical Approach
To tackle the hallucination issue, Google researchers devised a Selective Generation method that incorporates confidence scores alongside sufficient context signals. This method allows models to better assess whether to generate an answer or abstain, effectively reducing incorrect statements. The result is not only an improvement in the accuracy of AI responses but also a strategic approach to managing potential inaccuracies.
Implications for Content Creators and Publishers
This enhancement in RAG models carries profound implications for content creators and publishers. As AI-generated answers become increasingly reliant on the quality of available data, there is a growing responsibility to produce content that meets the standards for sufficient context. High-quality, contextually-rich content not only serves to assist AI but also engages users effectively, ensuring that their queries are met with reliable information.
The Future of AI and Contextual Understanding
The repercussions of Google’s research extend beyond immediate technical improvements. As AI systems become smarter, the understanding of context will play a crucial role in shaping future interactions between users and technology. With continued refinement in context recognition, the AI landscape may evolve to where hallucinations become a relic of the past, leading to more trustworthy and user-friendly AI solutions.
Conclusion
The quest for accurate AI responses is an ongoing journey marked by innovation and adaptation. Google's exploration into sufficient context marks a pivotal moment in AI evolution, providing a framework that not only mitigates errors but also fosters a deeper understanding of how contextual relevance shapes accuracy in automated responses. As this research unfolds, content creators and technology experts alike must stay informed on these developments to harness their full potential.
Write A Comment